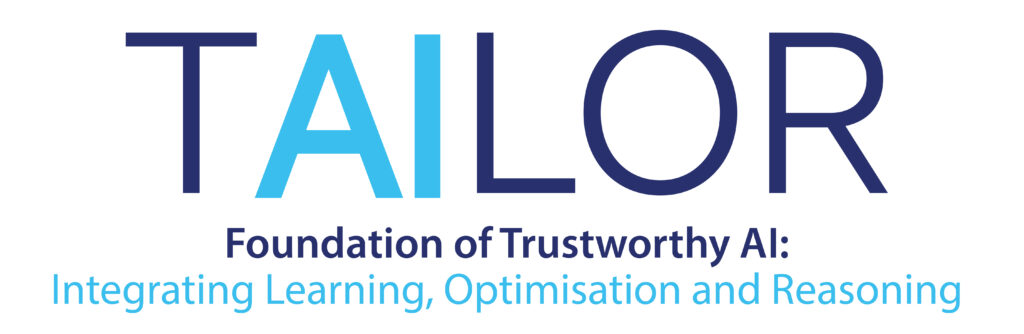
The TAILOR consortium is committed to demonstrate that research and innovation based on expertise, cooperation, and diversity can achieve the European vision of Human-Centered Trustworthy AI and make Europe the global role-model for responsible AI. Through clear objectives, TAILOR will bring together one of the largest networks of over 55 partners involved in AI research, leading to 190 + publications, and bringing us closer to this shared goal. You can find their main website here.

The quest for Trustworthy AI is high on both the political and the research agenda, and it actually constitutes TAILOR’s first research objective of developing the foundations for Trustworthy AI. It is concerned with designing and developing AI systems that incorporate the safeguards that make them trustworthy, and respectful of human agency and expectations. Not only the mechanisms to maximize benefits, but also those for minimizing harm. TAILOR focuses on the technical research needed to achieve Trustworthy AI while striving for establishing a continuous interdisciplinary dialogue for investigating the methods and methodologies to fully realize Trustworthy AI.
Learning, reasoning and optimization are the common mathematical and algorithmic foundations on which artificial intelligence and its applications rest. It is therefore surprising that they have so far been tackled mostly independently of one another, giving rise to quite different models studied in quite separated communities. AI has focussed on reasoning for a very long time, and has contributed numerous effective techniques and formalisms for representing knowledge and inference. Recent breakthroughs in machine learning, and in particular in deep learning, have, however, revolutionized AI and provide solutions to many hard problems in perception and beyond.
However, this has also created the false impression that AI is just learning, not to say deep learning, and that data is all one needs to solve AI. This rests on the assumption that provided with sufficient data any complex model can be learned.
Well-known drawbacks are that the required amounts of data are not always available, that only black-box models are learned, that they provide little or no explanation, and that they do not lend themselves for complex reasoning. On the other hand, a lot of research in machine reasoning has given the impression that sufficient knowledge and fast inference suffices to solve the AI problem. The well-known drawbacks are that knowledge is hard to represent, and even harder to acquire. The models are however more white-box and explainable. Today there is a growing awareness that learning, reasoning and optimization are all needed and actually need to be integrated. TAILOR’s second research objective is therefore to tightly integrate learning, reasoning and optimization. It will realize this by focusing on the integration of different paradigms and representations for AI.
A set of core work packages have been set out by the TAILOR project to overcome these challenges and reach our shared goal of in making Europe a global role-model in responsible AI. Each work package aims to solve cores tasks.
Projects - Work Packages
ALU-FR’s contributions
Our lab is highly involved with WP7 - AutoAI, specifically AutoML in the Wild:
Facilitate the usability of machine learning by non-machine-learning-experts who have data and a clear target to predict, but who are not familiar enough with machine learning to know which neural architecture or machine learning pipeline to use, and how to set its hyperparameters.
- We organized 4 conferences/workshops, an online course, the AutoML Fall School, in conjunction with several talks, helped us to connect with the end users of AutoML which amounted to over 2000 participants. Namely these were practitioners, researchers and students, enabling us to contextualize how AutoML is used in the wild and dictate further research aims and focuses.
- 4 papers were published in conjunction with other TAILOR partners aimed to reduce compute constraints and accelerate research, each at high impact journals/conferences that allow fast and standardized benchmarks for all common branches of AutoML, notably HPO, NAS and Algorithm Configuration, each of which are fields aimed on reducing the users prior knowledge requirements.
- Expert domain knowledge is still invaluable and can be used to warm-start optimization, for which 1 further paper demonstrates that any user knowledge can, and should, be incorporated, allowing first-time users to domain-experts to benefit from these advancements.
- Meta-learning and dynamic algorithm configurations are core to bringing success to the world of Reinforcement Learning in the real world. To this end, we published a paper on how algorithms can benefit from both context and meta-learning!
- The tools we created during this process has directly benefited from these advancements and are now seeing over 4000+ downloads daily, as of the time of writing.
Partners
There are too many partners to list but you can find a full list here. You can jump to the websites of some of our partners for WP7 AutoAI with the images below!