Active Funded Projects
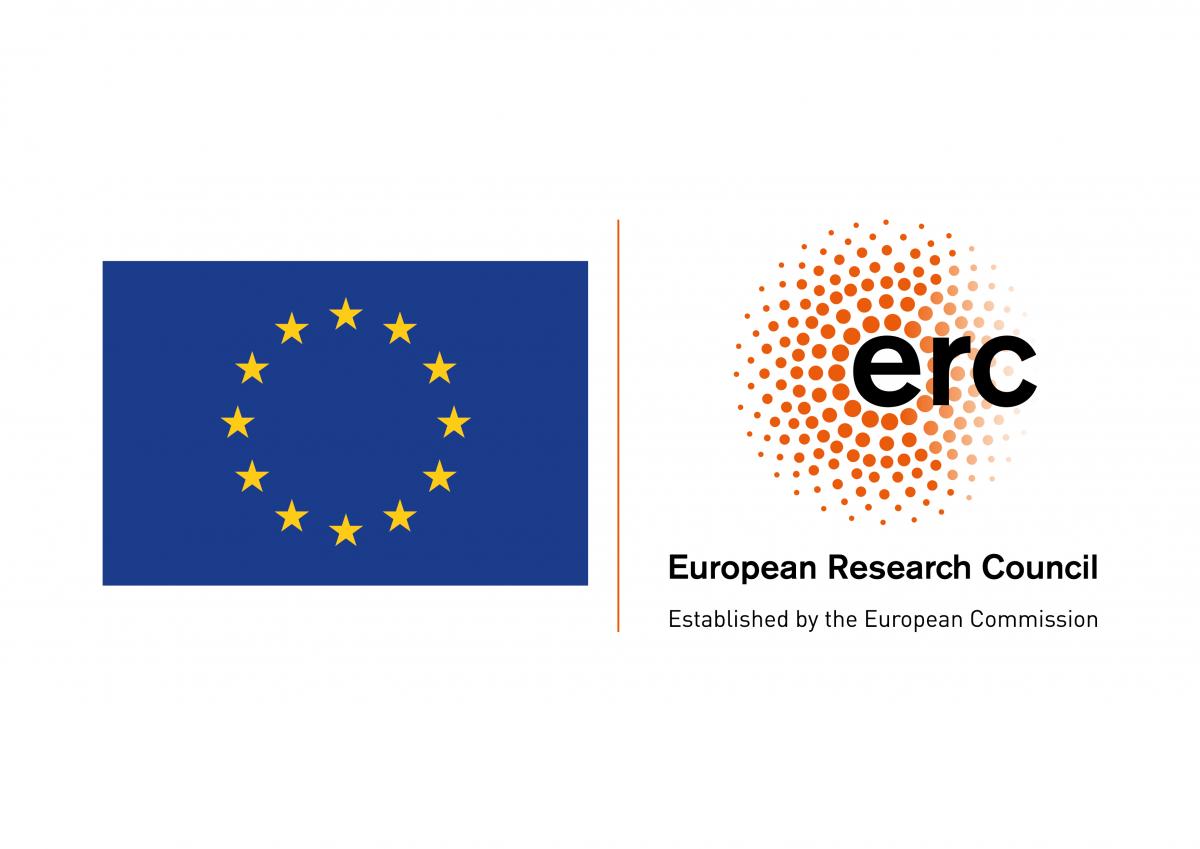
ERC Consolidator Grant: Deep Learning 2.0
Funding Agency: European Research Council
Funding amount: 2M Euros, for basic research
Funding period: 01.05.2022 - 30.04.2027
With Deep Learning 2.0, the European Research Council funds our basic research on the foundations of the next generation of deep learning. While deep learning replaced domain-specific hand-crafted features with features that are learned for the particular task at hand, DL 2.0 takes deep learning to the next level and also (meta-)learns other hand-crafted elements of the deep learning pipeline. This can lead to great performance improvements, realizing that, throughout the history of AI, handcrafted components have been successively replaced by better-performing, automatically learned ones. Importantly, this also allows to take into account various constraints and objectives of the problem at hand, such as robustness, hardware requirements, ecological footprint, latency, interpretability, training cost, uncertainty estimation, and algorithmic fairness, positioning this approach perfectly to achieve Trustworthy AI. In general, ERC grants are Europe’s most prestigious funding instrument, focused purely on an excellence-based approach to science. As stated in a recent Nature article, the ERC has helped Europe to “surpass the United States in terms of the most-cited scientific publications” and is “recognized as the best in the world in the way it supports fundamental research”.
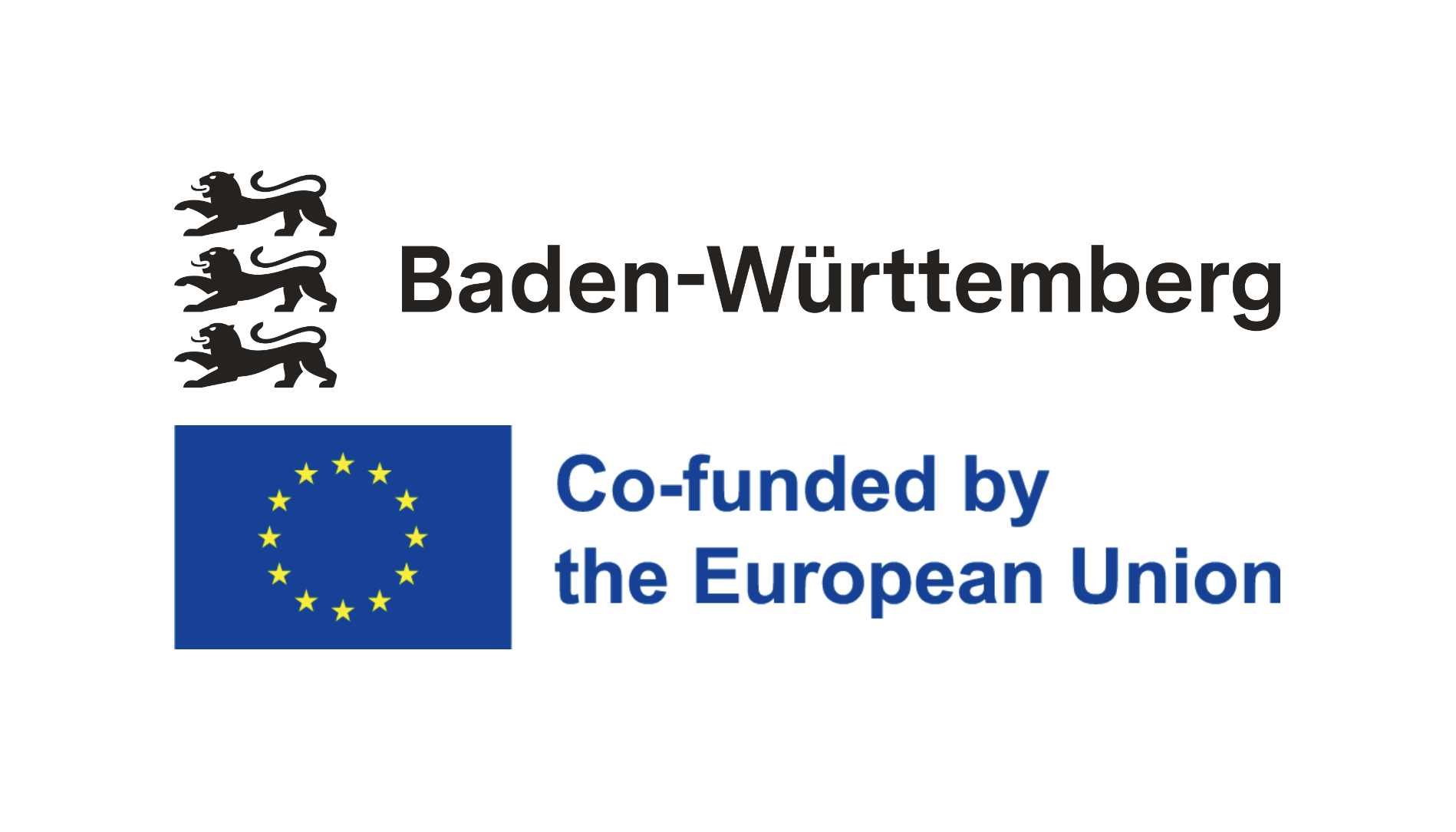
Deep Learning Cluster
Funding Agency: Deutsche Forschungsgemeinschaft (DFG, German Research Foundation) under grant number 539134284, through EFRE (FEIH_2698644) and the state of Baden-Württemberg
The Deep Learning Cluster expands and modernizes the existing infrastructure to advance fundamental research in deep learning at the University of Freiburg. By integrating high-performance, energy-efficient GPU and CPU computing units, along with enhanced storage capacities, the cluster will enable the development of novel, transformative machine learning methods. These methods will support a wide range of research areas, including generative language models, multimodal models combining language and vision, autonomous driving, robotics, bioinformatics, medical image processing, and energy-efficient machine learning. A central focus of this initiative is to promote sustainable and energy-conscious AI development, laying the groundwork for a greener digital future.
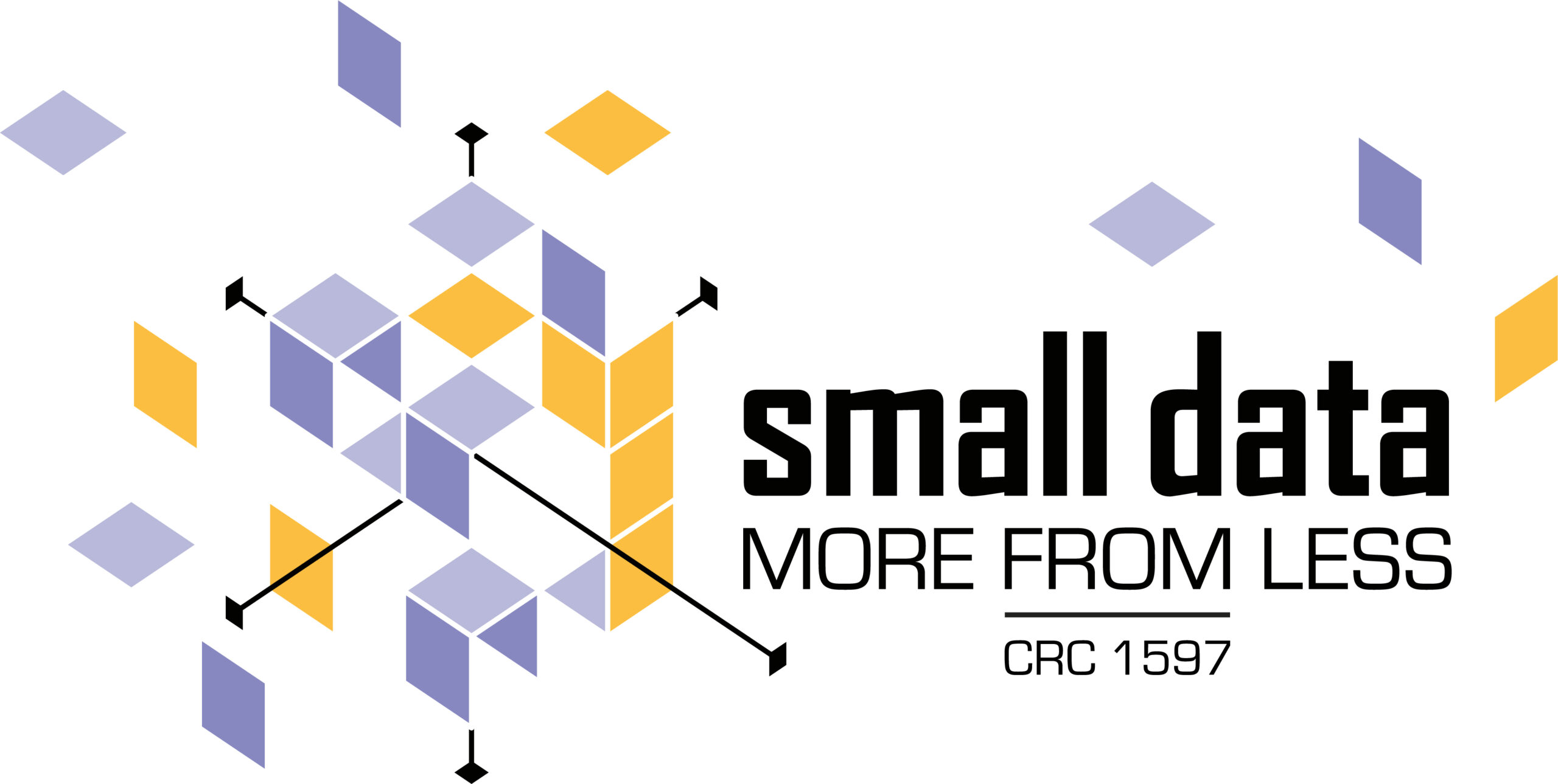
Learning fast and efficient hyperparameter control for deep reinforcement learning on small datasets
Funding Agency: Deutsche Forschungsgemeinschaft (DFG, German Research Foundation)
Deep reinforcement learning (RL) algorithms are a powerful class of methods for optimizing sequential decision making and control problems, and are the driver behind many real-world applications. We hypothesize that deep RL applications can considerably benefit from a focus on hyperparameter tuning. Specifically, we aim to develop automated techniques for setting the hyperparameters in a sample-efficient manner for small datasets, thus reducing uncertainty. We will create appropriate benchmarks and then investigate the performance of currently existing hyperparameter optimization (HPO) methods, to then develop automated approaches. This will include a dynamic meta-level control with the use of a robust hyperparameter transfer for online HPO for offline RL and neural architecture search (NAS).
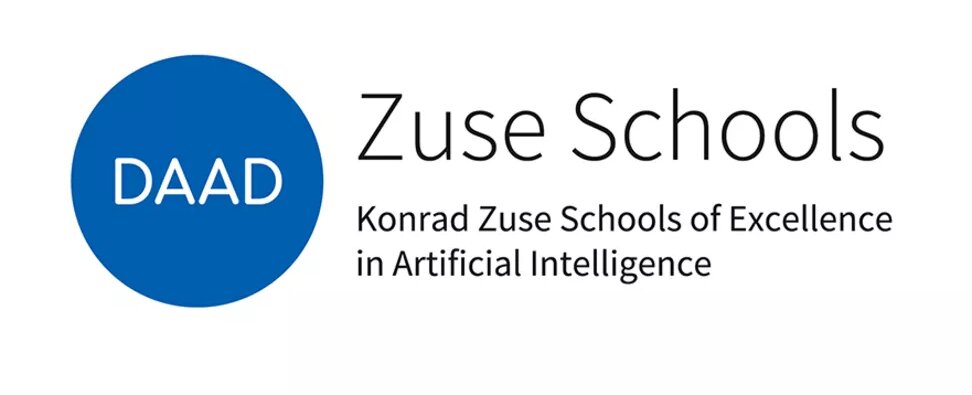
Zuse School ELIZA
Funding agency: Federal Ministry of Education and Research (BMBF) through the German Academic Exchange Service (DAAD) 2022-2027.
The Konrad Zuse School of Excellence in Learning and Intelligent Systems (ELIZA) is a graduate school in the field of artificial intelligence. ELIZA’s research and training activities focus on four main areas: the basics of machine learning — including ML-driven fields like computer vision or robot learning —, machine learning systems, applications in autonomous systems, as well as trans-disciplinary applications for machine learning in other scientific fields, from life sciences to physics.
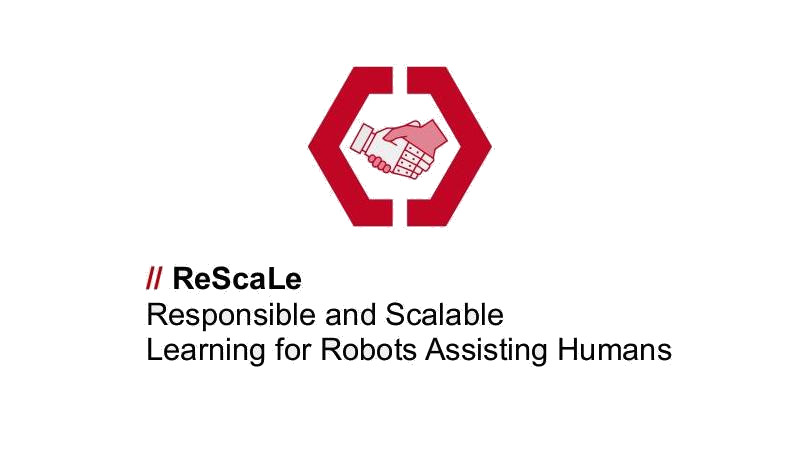
ReScaLe
Funding agency: Carl-Zeiss-Stiftung
The ReScaLe Project has multiple goals. To begin with, robots should be able to learn from observing demonstrations given by humans without having to retrain them completely. Robots should also be able to transfer policies to new environments by meta-learning on a set of environments. At the same time, the focus is on responsible development of AI and AI-based robotic systems that are based on human rights. Finally, the successful mutual transfer of knowledge from scientific to non-scientific actors and vice versa needs to be ensured.

Neural Architectures for Autonomous Driving
Funding agency: Bosch
Bosch and the University of Freiburg have initiated a three-year research collaboration to advance transparent, scalable AI for autonomous driving. A key focus is on neural architecture search (NAS), aiming to automatically design efficient, adaptable AI models for complex driving tasks. Ten PhD students will work across three labs to develop interpretable systems that integrate perception, planning, and decision-making. The project combines theoretical research with practical testing on Bosch autonomous vehicles to ensure real-world impact.
Completed Projects
ERC Grant
Data-Driven Methods for Modelling and Optimizing the Empirical Performance of Deep Neural Networks (BeyondBlackBox)
Entirely fundamental research, funded by the European Research Council with 1.5M Euro
Emmy Noether project
Advanced Methods for Automated Optimization and Modeling of the Empirical Performance of Highly Parameterized Heuristic Algorithms
Entirely fundamental research, funded by the DFG
Google Faculty Research Award
Efficient Neural Architecture Search
Entirely fundamental research
DFG project
Auto-Tune: Structural optimization of Machine Learning frameworks for large datasets
Entirely fundamental research, funded by the DFG
Part of the DFG Schwerpunktprogramm 1527 on "Autonomous Learning"
EU project
RobDREAM
Research in a consortium of industrial and academic partners, funded by the European Commission
Various subprojects in the Excellence cluster
BrainLinks-BrainTools
Entirely fundamental research, funded by the DFG
Bosch - Uni Freiburg Collaboration on AutoML
This project focuses on foundational research on AutoML, including neural architecture search, hyperparameter optimization and learning to learn. Even though it is an industrial collaboration, we devised the entire research plan alone, focused on foundational research and explicitly did not take into account which parts may be interesting for Bosch's business interests. The goal is simply to carry out world-class research in AutoML, publish at top venues, and open-source our research.
TAILOR - Foundations of Trustworthy AI – Integrating Reasoning, Learning and Optimization
The TAILOR consortium is committed to demonstrate that research and innovation based on expertise, cooperation, and diversity can achieve the European vision of Human-Centered Trustworthy AI and make Europe the global role-model for responsible AI. Through clear objectives, TAILOR will bring together one of the largest networks of over 55 partners
involved in AI research, leading to 193 publication, and bringing us closer to this shared goal.
KI: I4C - Intelligence for Cities
The project "Intelligence for Cities" is a joint effort between the Albert-Ludwigs-University of Freiburg, the Freiburg-based Fraunhofer Institutes ISE and IPM and the Sustainability Centre Freiburg. The project is funded by the German Federal Ministry for the Environment, Nature Conservation, Nuclear Safety and Consumer Protection (BMUV) through the funding initiative "KI Leuchttürme". The goal of the project is to develop a 3D-model of urban space including meteorological variables through a process chain from data collection, analysis and environmental forecasting to concrete measures. The project aims to provide an AI-toolbox under open-source-license as a starting point for future research endeavors and to support local city planning.
RenormalizedFlows
The interdisciplinary project RenormalizedFlows combines neuroscience, computer science, statistical physics and medicine to deliver fundamental advances in the transparency and interpretability of deep neural networks. These networks consist of a cascade of many layers of neurons that break down incoming data, step by step, into its underlying causes. However, this decomposition is so complex that it is not directly comprehensible for a human being. In this project we aim to achieve concrete progress in explainable and transparent deep learning, by building upon recent developments in the field of invertible deep networks, neural architecture search, meta-learning and hyperparameter optimization.